Understanding CAD Rads: Innovations in Radiology
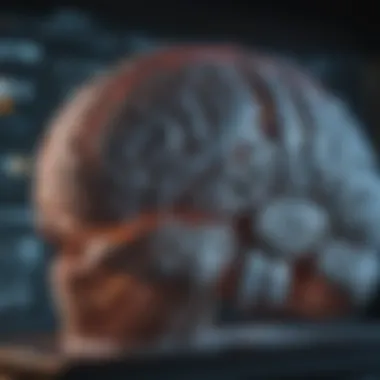
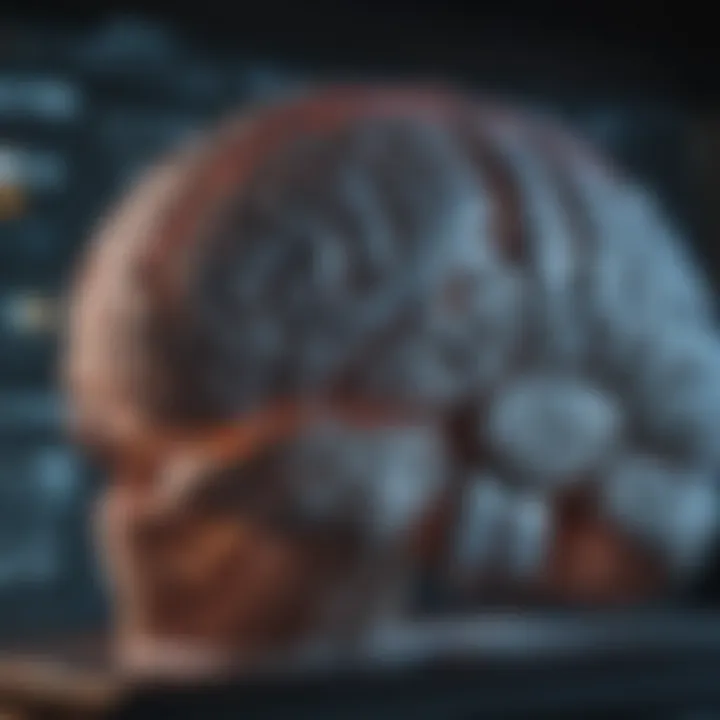
Intro
The field of radiology has significantly advanced with the integration of technology. One of the groundbreaking innovations is Computer-Aided Design in radiology, often termed CAD Rads. This advanced tool is crucial in enhancing diagnostic procedures. It assists radiologists in analyzing medical images with higher accuracy and efficiency. Understanding the functioning and benefits of CAD Rads can open doors to improving patient care in various medical environments.
In this article, we will explore the fundamental principles that govern CAD Rads, along with the evolving technologies that accompany it. The focus will also be on the practical applications of this technology and the significant role it plays in the workflow of medical imaging professionals. Moreover, we will discuss the challenges that CAD Rads faces in clinical settings and the implications these challenges hold for the future of radiology.
Establishing a comprehensive understanding of CAD Rads is essential, as it not only contributes to better diagnostic accuracy but also makes the workflow more streamlined. We aim to provide insights into how CAD Rads is shaping modern radiology and what it means for future advancements in the field.
Prelims to CAD Rads
Computer-Aided Design in radiology, commonly referred to as CAD Rads, marks a transformative shift in the field of medical imaging. This section aims to elucidate the significance of CAD Rads and its implications in today's healthcare environment. The integration of CAD systems stands at the intersection of technology and medicine, promising enhancements in diagnostic precision and operational efficiency.
The essence of CAD Rads lies in its ability to assist radiologists in interpreting imaging data. By leveraging advanced algorithms and sophisticated imaging techniques, CAD systems can detect abnormalities that may elude the human eye. This critical aspect underscores the necessity for education and understanding among professionals who utilize these tools daily.
Moreover, CAD Rads offers several benefits:
- Increased Diagnostic Accuracy: The system's ability to flag potential issues enhances the likelihood of correct diagnoses.
- Workflow Improvement: Automating time-consuming tasks allows radiologists to focus on complex cases that demand human expertise.
- Support for Training and Research: CAD tools provide valuable resources for educational purposes and ongoing research initiatives, bridging gaps in knowledge.
Despite its advantages, the deployment of CAD Rads does not come without considerations. Issues such as technical limitations, regulatory hurdles, and ethical implications must be carefully navigated. Understanding these factors is crucial for effective implementation and utilization of CAD systems in clinical practice.
As we delve into the specifics of CAD Rads, it becomes evident that this technology represents not just a tool, but a comprehensive framework that can reshape the landscape of radiology as we know it.
Defining Computer-Aided Design in Radiology
Computer-Aided Design has evolved over time to serve various industries, including architecture and manufacturing. In radiology, CAD serves a unique function: it aids healthcare professionals in the analysis and interpretation of medical images. Essentially, CAD algorithms analyze imaging data and provide a set of prioritized recommendations or alerts regarding potential anomalies.
These systems bring significant computational power and speed to the diagnostic process, processing large sets of image data with precision. By presenting these insights to radiologists, CAD helps to enhance human decision-making, thereby increasing the reliability of diagnoses.
Historical Context of CAD Rads
The journey of CAD in radiology dates back several decades. The inception of these systems can be traced to the 1980s, when researchers began experimenting with image processing techniques. Initial applications focused on mammography, aiming to improve the detection of breast cancer. With time, the technology matured, incorporating advancements in computer vision and machine learning.
Over the years, the increasing digitization of medical imaging paved the way for broader acceptance and integration of CAD systems. By the early 2000s, regulatory bodies began to approve CAD tools for various imaging modalities. This proactive engagement highlighted the potential of CAD to change conventional practices in radiology.
Today, CAD Rads stands as a testament to technological innovation, reflecting a need for continuous improvement in diagnostic capabilities. The evolution showcases a relentless pursuit of enhancing patient care through precise imaging and analysis.
Fundamental Principles of CAD Rads
The fundamental principles of Computer-Aided Design in radiology, or CAD Rads, serve as the backbone of its application and significance in modern medical imaging. Understanding these principles is vital, as they clarify how CAD enhances diagnostic processes and streamlines radiological workflows. CAD Rads not only assists practitioners in detecting abnormalities but also supports them in making informed decisions based on data that is analyzed through sophisticated algorithms.
How CAD Rads Works
CAD Rads operates on a model of data processing that relies heavily on software algorithms to analyze images from various imaging modalities. Primarily, it involves several intertwined steps:
- Image Acquisition: The initial phase involves capturing medical images through modalities like X-rays, CT scans, or MRIs. These images are then digitally processed for analysis.
- Image Processing: Advanced algorithms scan the acquired images to enhance features of interest. The focus generally lies on identifying potential abnormalities such as tumors or fractures.
- Detection and Analysis: Here, the core CAD algorithms apply their learning from vast datasets to detect anomalies. They highlight suspected areas for radiologists, which is pivotal in enhancing diagnostic accuracy.
- Post-Processing: The system allows practitioners to review findings against clinical guidelines or databases to support decision-making.
Overall, the relationship between image processing and subsequent analyses establishes a feedback loop where the accuracy of results improves as more cases are assessed. This dynamic is crucial for reducing human error and enhancing patient outcomes.
Key Components of CAD Systems
A successful CAD system incorporates several essential components to function efficiently. These components work together to create a robust platform for diagnostic support:
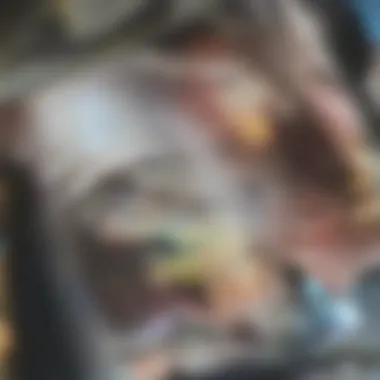
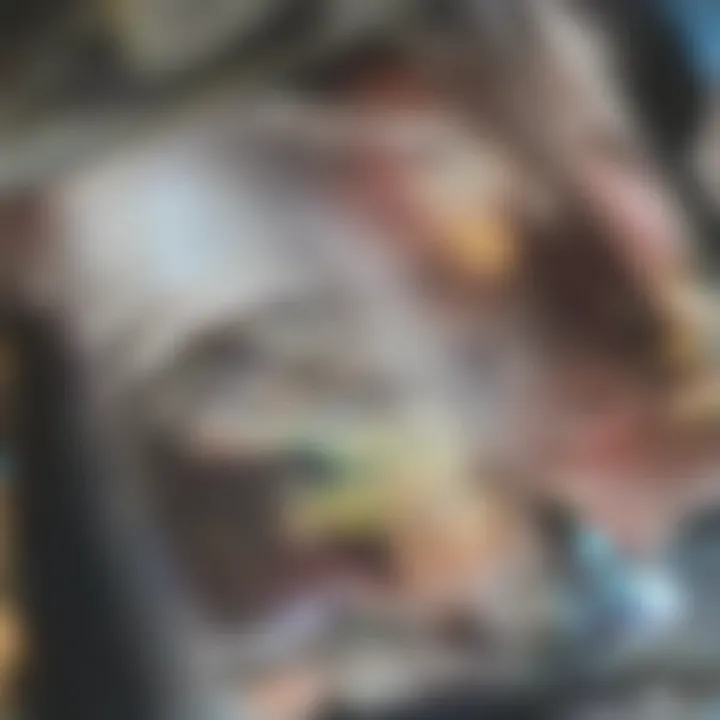
- Image Interpretation Engine: This is the heart of a CAD system. It contains the algorithms and methods for image processing and data behavior.
- User Interface: A streamlined and intuitive interface enables radiologists to interact easily with the system. This component is crucial because it allows for efficient and accurate usage of the CAD tools.
- Database Management: Storing and retrieving vast amounts of imaging data and relevant patient information is necessary. A strong database management system supports effective data analysis and reporting.
- Feedback Mechanism: Most CAD systems have a mechanism to learn from user interactions. This component improves the algorithm's performance over time, making it more reliable for repeated use.
Thus, each of these components plays a significant role in ensuring that CAD systems function optimally and support radiologists in providing accurate diagnoses and treatment plans.
"CAD Rads technologies provide a sophisticated layer of analysis that complements human capabilities, which is essential in modern healthcare."
These principles of CAD Rads are not just theoretical; they actively shape the landscape of radiology, influencing how healthcare professionals engage with diagnostic imaging.
Technological Advancements in CAD Rads
Technological advancements are crucial for the evolution of Computer-Aided Design in radiology (CAD Rads). These innovations redefine how images are processed, analyzed, and interpreted. To appreciate the full impact of CAD Rads, understanding the pivotal roles played by machine learning, artificial intelligence, and integration with various imaging techniques is essential.
Machine Learning and Artificial Intelligence
Machine learning and artificial intelligence (AI) represent significant strides in CAD Rads. These technologies enhance the ability to detect abnormalities in medical images. With algorithms capable of learning from vast datasets, machine learning models improve over time. This means they can identify patterns that may be challenging for human eyes to perceive. The implementation of AI allows for quicker analysis, which can be crucial in time-sensitive diagnoses.
Machine learning techniques like convolutional neural networks (CNNs) are especially beneficial in image classification tasks. They identify features in images, leading to more accurate diagnostic results. For instance, in oncology, these technologies help detect tumors with increased sensitivity and specificity. Incorporating AI in CAD systems can significantly reduce false positives and negatives.
"The integration of machine learning leads to a more informed decision-making process in radiology."
Furthermore, machine learning models can continuously learn from new data. This ability to adapt to new information makes the systems dynamic and constantly improving. With AI, radiologists can prioritize their workload based on urgency, thus enhancing overall efficiency in clinical settings.
Integration with Imaging Techniques
Integrating CAD Rads with various imaging techniques is another critical aspect of recent advancements. Modalities like Magnetic Resonance Imaging (MRI), Computed Tomography (CT), and ultrasound benefit from CAD systems. Each imaging technique presents unique challenges, and CAD Rads aims to address these through tailored solutions.
For example, when using CT scans for pulmonary assessments, CAD systems aid in identifying nodules and lesions. The algorithms analyze thousands of images rapidly and accurately. Similarly, combining CAD with MRI enhances the assessment of soft tissue, benefiting the diagnosis in neurology.
Moreover, the interoperability of CAD systems with imaging devices is increasingly common. This synergy allows for seamless workflows, minimizing the time from image acquisition to interpretation. As imaging technologies advance, the need for sophisticated CAD systems that can integrate efficiently becomes more essential.
Practical Applications of CAD Rads
The realm of Computer-Aided Design in radiology extends well beyond theoretical discussions. The practical applications of CAD Rads represent its real-world impact on diagnostic processes across various medical disciplines. By leveraging CAD technologies, healthcare providers can enhance the speed and accuracy of imaging interpretations, promoting better patient outcomes. This section elucidates how CAD systems are being utilized in different specialties, optimizing diagnostic capabilities and streamlining workflows.
In Oncology
In oncology, CAD Rads is pivotal for detecting and characterizing tumors. CAD tools analyze imaging data from modalities like mammography and CT scans, identifying regions that might require further investigation. For instance, in breast cancer detection, CAD algorithms can highlight microcalcifications and masses that may be overlooked by human eyes. This early detection is vital, as it often leads to more effective treatment options. Moreover, studies indicate that the use of CAD can substantially increase sensitivity rates, leading to earlier diagnoses and improved survival rates in patients.
In Neuroscience
In the field of neuroscience, CAD Rads enhances the evaluation of brain imaging studies. The complexity of neurological conditions requires precise analysis of MRIs and CT scans. CAD systems can assist in identifying subtle changes in brain morphology associated with various conditions such as Alzheimer’s disease and multiple sclerosis. By automating the detection of lesions or anomalies, these systems reduce the time radiologists spend interpreting images. As a result, healthcare providers can make faster decisions, facilitating timely interventions that are crucial in managing neurological disorders.
In Cardiovascular Imaging
Cardiovascular imaging similarly benefits from CAD technology. With an increasing prevalence of heart diseases, CAD Rads offers tools for the assessment of coronary artery conditions through CT angiography or echocardiograms. CAD algorithms help in the quantification of stenosis and other cardiac abnormalities. They provide accurate measurements that aid in determining the severity of blockages. Additionally, CAD can enhance the detection of conditions like aortic aneurysms by meticulously analyzing imaging data to identify risk factors. Ultimately, this technology contributes to improved management plans tailored to individual patient needs.
"The integration of CAD Rads in various medical fields shows promise in altering the landscape of diagnostic imaging, presenting a future where efficiency and accuracy are substantially improved."
Impact on Diagnostic Accuracy and Workflow
The integration of Computer-Aided Design (CAD) systems into radiology presents substantial implications for both diagnostic accuracy and workflow efficiency. As radiologists face increased volumes of imaging studies, CAD technology serves as a critical ally in improving detection rates and expediting processes. By analyzing vast datasets, CAD brings forth insights that may not be readily apparent to the human eye, thus enhancing overall diagnostic confidence.
Enhancing Detection Rates
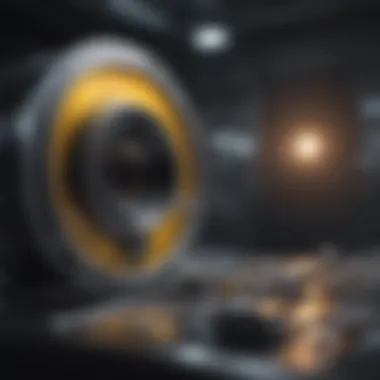
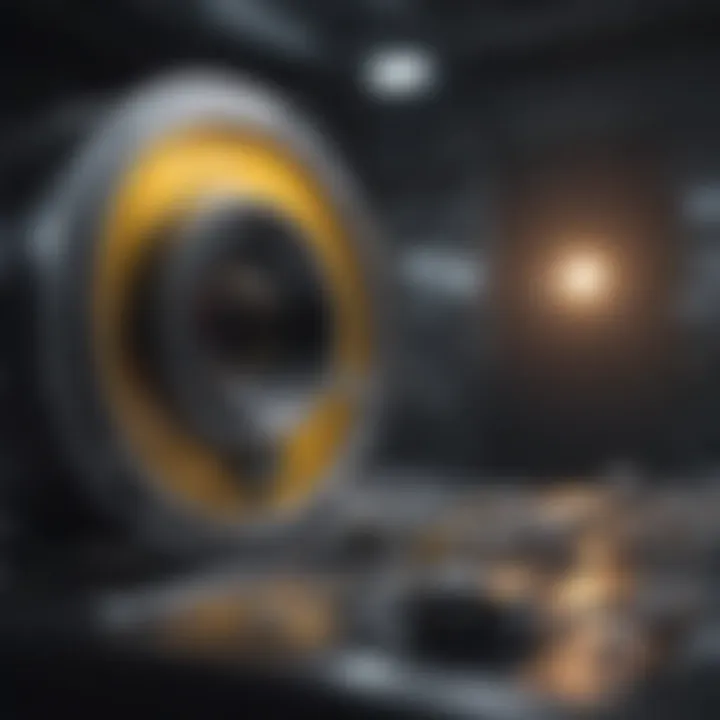
Detection of abnormalities is a fundamental aspect of radiology. CAD systems are specifically designed to assist radiologists in identifying significant findings, leading to improved detection rates across different medical scenarios. For instance, studies have shown that implementing CAD in mammography can increase the sensitivity of breast cancer detection. This is crucial because early detection significantly impacts treatment outcomes.
Several factors contribute to the enhanced detection capability of CAD:
- Algorithmic Precision: CAD algorithms utilize advanced machine learning techniques to analyze images. This allows for the recognition of patterns that align with various pathologies.
- Supplementary Detection: CAD acts as a second set of eyes. Even in skilled hands, subtle findings can be missed. CAD systems flag these potential issues for radiologists, facilitating a thorough review.
- Feedback Loop: Continuous learning from previous cases allows CAD systems to evolve. As radiologists interact with the system, these interactions help improve future accuracy.
In a study published in a leading radiology journal, hospitals utilizing CAD reported a marked increase in cancer detection rates, confirming the validity and effectiveness of these systems.
"CAD systems are not meant to replace radiologists but to empower them with advanced tools for better patient outcomes."
Streamlining Radiological Processes
Efficiency in workflow is essential for healthcare facilities to optimize resources and improve patient care. CAD solutions contribute to streamlining radiological processes through various mechanisms.
- Automation of Routine Tasks: Tasks such as image segmentation and preliminary analysis can be automated by CAD systems. Radiologists can then focus on interpreting results instead of laboriously processing images, leading to quicker diagnoses.
- Prioritization of Cases: CAD systems can triage imaging studies based on the likelihood of critical findings. This allows for prioritizing urgent cases, ensuring that patients receive timely attention.
- Data Management: CAD systems often come with integrated data management capabilities. This feature minimizes the complexity of managing multiple imaging studies and contributes to smoother case workflows.
The streamlined processes facilitated by CAD also enable radiologists to handle a higher number of cases without compromising quality. As such, patients benefit from reduced wait times for results and subsequent interventions.
In summary, CAD systems redefine the landscape of radiology, enhancing detection rates and overall operational workflow. As technology continues to evolve, the potential for CAD to further revolutionize these aspects remains promising.
Challenges and Limitations of CAD Rads
The integration of Computer-Aided Design in radiology offers notable benefits, but it is essential to recognize the challenges and limitations associated with its application. Understanding these challenges is crucial for practitioners and stakeholders in the healthcare field. They impact not only how CAD Rads is utilized but also its effectiveness and overall acceptance in clinical settings.
Technical Limitations
One of the primary technical limitations involves the accuracy and reliability of CAD systems. Although CAD tools are developed to assist radiologists in identifying abnormalities, they are not infallible. The underlying algorithms can only process the data they are trained on. If a system lacks sufficient and diverse training data, its ability to detect various conditions may be compromised. Additionally, some CAD systems can struggle with complex imaging scenarios, such as overlapping structures or noise artifacts.
Inconsistency in imaging quality can further complicate the process. Variations in equipment, patient positioning, and even the radiologist’s technique may lead to inconsistent outcomes when utilizing CAD tools. This variability can affect the confidence radiologists have in the system recommendations, resulting in hesitation to fully rely on such technologies.
Some other technical limitations include:
- Limited adaptability across different imaging modalities.
- Inability to interpret non-standardized images.
- High computational costs associated with advanced machine learning algorithms.
These factors underline the need for robust systems and continuous improvement in the algorithms used in CAD Rads.
Regulatory and Ethical Considerations
Regulatory and ethical considerations are imperative when discussing the limitations of CAD Rads. Regulatory bodies, such as the Food and Drug Administration (FDA), carefully monitor the development and deployment of CAD systems. Compliance with regulations is crucial to ensuring patient safety, especially in the context of diagnostic tools. New technologies must undergo rigorous testing and validation before widespread use.
Moreover, ethical concerns around patient privacy and data security cannot be overlooked. CAD systems often work with sensitive medical data, which raises questions about data handling, storage, and sharing practices. If these systems do not adhere to strict data privacy guidelines, there can be significant repercussions for patients and institutions alike.
There are additional ethical issues related to decision-making. With CAD tools providing recommendations, there is a danger that reliance on technology might lessen critical human judgment. Radiologists might lean too heavily on system outputs rather than applying their expertise, possibly diminishing the quality of care.
"A careful balance must be struck to ensure that CAD tools augment rather than replace the radiologist's expertise."
Thus, addressing regulatory and ethical considerations is crucial for fostering trust in CAD Rads and ensuring that these systems enhance patient outcomes rather than compromise them.
Future Directions in CAD Rads
Future directions in Computer-Aided Design in radiology (CAD Rads) are of great importance as they shape the landscape of medical imaging and diagnostic practices. As technology evolves, CAD Rads has the potential to transform not only how images are interpreted but also how radiologists interact with patient data. Enhancements in algorithms and the integration of advanced technologies promise to improve diagnostic accuracy significantly. This section explores the emerging trends in research and proposed innovations that may drive these advancements.
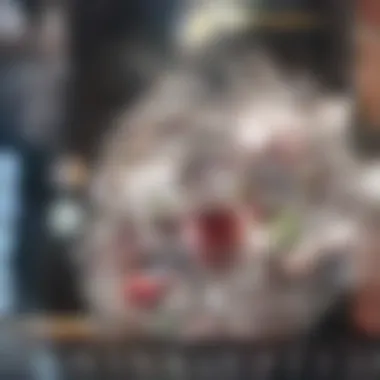
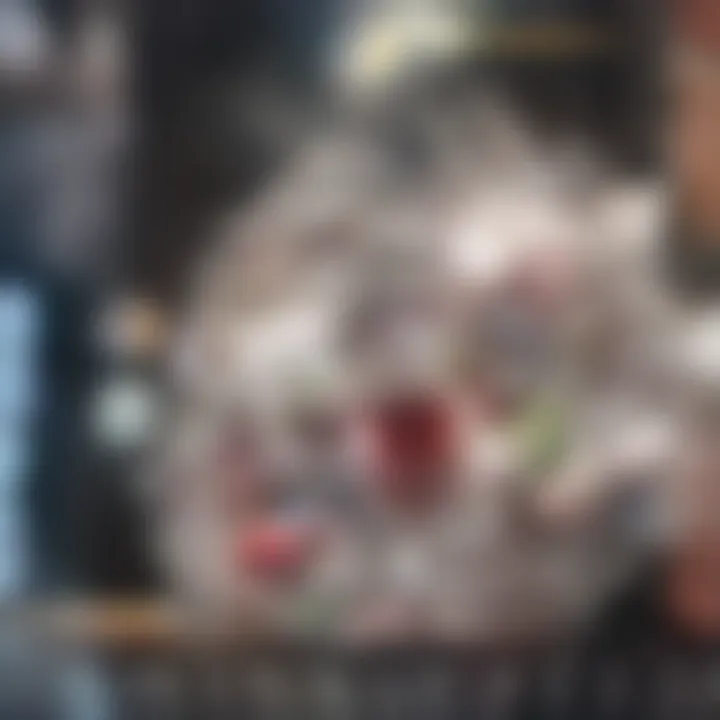
Emerging Research Trends
The realm of CAD Rads is currently experiencing profound research activity. Key trends include the development of more robust machine learning models that improve upon traditional algorithms. Recent studies focus on deep learning techniques. These techniques have shown promise in enhancing the precision of image analysis. Researchers are also examining how multi-modal data integration, which combines data from different imaging modalities such as MRI and CT scans, can lead to more comprehensive diagnostics.
Further, attention is being given to the ethical implications of using artificial intelligence in radiology. Investigating the bias in algorithms and ensuring equitable access to technology are essential elements of ongoing research. Notably, collaborative projects between medical institutions and tech companies are becoming increasingly common. These partnerships aim to translate innovative research directly into clinical practice, which could result in improved patient outcomes.
Proposed Innovations in Technology
Looking ahead, various innovations in technology promise to advance CAD Rads further. One significant area is the development of real-time processing systems. This system will allow radiologists to receive immediate feedback on image interpretations. Such innovations can streamline workflow and reduce errors in diagnostics.
Additionally, the integration of cloud computing with CAD systems can facilitate easier access to large datasets across different institutions. This can enhance collaborative analysis and foster data sharing, which is critical for research and improving diagnostic techniques.
The possible introduction of augmented reality (AR) tools in CAD Rads is also noteworthy. AR could provide an interactive environment for radiologists, allowing them to visualize complex data in a more intuitive manner.
"The evolution of CAD technology is not just about improving accuracy; it is about changing the way radiologists work and interact with their data.”
In summary, the future of CAD Rads holds considerable promise. Emerging research trends, along with groundbreaking technological innovations, have the potential to redefine radiological practices. These advancements are crucial for enhancing diagnostic capabilities, ensuring reliable patient care, and addressing challenges in the medical imaging landscape.
Case Studies in CAD Rads Implementation
In the evolving landscape of medical imaging, case studies provide essential insights into how Computer-Aided Design (CAD) can be successfully implemented in clinical practices. These real-world examples illuminate both the advantages and challenges of integrating CAD systems in radiology. The importance of analyzing these case studies lies in understanding how CAD Rads addresses specific diagnostic needs, enhances workflow efficiency, and adjusts to unique clinical environments.
Successful Integration in Clinical Settings
The adoption of CAD Rads in healthcare facilities has proved to be transformative in many assessments. Effective integration often includes collaboration among radiologists, engineers, and IT teams, thereby creating a multifaceted approach to patient care. For example, in a hospital setting, integrating CAD software with existing imaging equipment can increase detection rates significantly.
- Workflow Improvement: The streamlined processing abilities of CAD systems help radiologists to review images quickly. This leads to timely diagnostics, which is crucial for patient care.
- Risk Reduction: Employing CAD enhances the accuracy of detecting abnormalities. It can potentially reduce the number of false negatives and missed cases in screening procedures.
- Personalized Learning: Healthcare institutions benefit from CAD implementations that tailor their functionalities to specific disease patterns prevalent in their patient population. This localization can enhance diagnostic relevance.
Such practical successes highlight the need for informed choices regarding the selection and adoption of CAD technologies in various clinical settings.
Lessons Learned from Failed Implementations
Despite notable successes, not all CAD implementations are successful. Understanding the reasons behind failed implementations is crucial to avoiding similar pitfalls in the future. Several common factors lead to these challenges:
- Poor User Training: Insufficient training on CAD software can hinder its effective use. Radiologists must be educated not just about the technology but also how to interpret and trust its output.
- Integration Issues: When CAD systems do not seamlessly integrate with current imaging modalities, it can create inefficiencies. For example, a mismatch between the timeline of generating images and CAD processing can delay diagnoses.
- High Expectation Management: Overestimating CAD capabilities can lead to disappointment. It should not be viewed as a replacement for human expertise, rather as a supportive tool.
Learning from these lessons remains key to ensuring that future CAD deployments are more fruitful. Investing time in the development of robust training programs is essential, as is engaging stakeholders early in the implementation process.
"Technology alone cannot solve the challenges we face; a comprehensively thought-out approach is necessary to ensure success."
The End
The conclusion of this article encapsulates the essential findings concerning Computer-Aided Design in radiology, also referred to as CAD Rads. Throughout the discussions, we focused on how CAD Rads enhance diagnostic accuracy and streamline workflow within radiological practices. In an era where medical technology advances rapidly, the integration of CAD systems becomes crucial for improving patient outcomes and facilitating sophisticated analysis of medical images.
Summarizing Key Insights
When examining CAD Rads, several key insights surface:
- Enhancement of Diagnostic Accuracy: CAD systems contribute significantly to the early detection of anomalies, reducing the number of missed diagnoses.
- Workflow Efficiency: Integration of CAD into radiological practice helps prioritize cases, thereby it reduces the overall time taken for image analysis.
- Challenges Addressed: The article also highlights the challenges facing CAD systems, such as technical limitations and regulatory hurdles that must be navigated to realize their full potential.
- Future Prospects: Ongoing research and real-world applications bear witness to the evolving landscape of CAD, promising more refined tools for radiologists.
Understanding these points forms a solid foundation on which stakeholders can base future advancements in CAD Rads.
The Path Forward for CAD Rads
Looking ahead, several paths emerge for the development and implementation of CAD Rads:
- Increased Integration with AI: As artificial intelligence continues to evolve, expect deeper integration with CAD systems, enhancing the capability of detecting subtle abnormalities in medical images.
- Regulatory Adaptation: Regulatory bodies must adapt to these technologies by creating frameworks that ensure safety and efficacy without stifling innovation.
- User Training and Familiarization: Ongoing training programs for medical professionals are vital. Familiarizing radiologists with new CAD tools is essential for maximizing their benefits.
- Research and Collaboration: Continuous research initiatives and collaboration between technology developers and healthcare professionals are necessary to refine existing systems and explore new methodologies.
In summary, CAD Rads stands at the forefront of enhancing radiology. Its future relies on technological advancements, supportive regulatory environments, and comprehensive training for medical practitioners.