Correlation Data Sets: Understanding Scientific Relationships
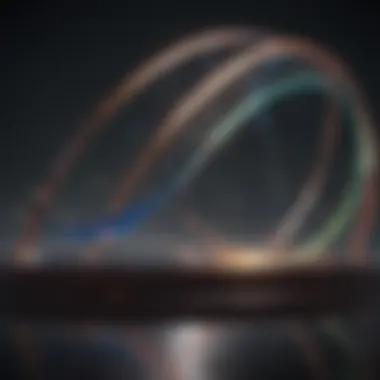
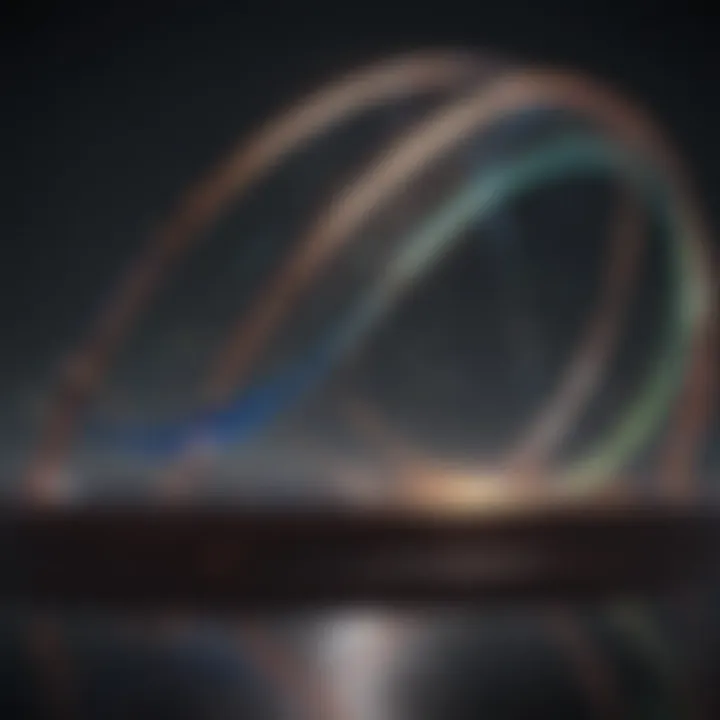
Intro
In scientific research, understanding how variables relate is crucial. Often, the relationships between different elements can unveil significant patterns or trends that aid further study. Correlation data sets serve as a foundational tool for scientists across various fields, helping them decipher these intricate relationships.
By assessing the strength and direction of relationships within data, researchers can form hypotheses that steer their investigations. It's not merely about identifying if a relationship exists, but understanding its depth and nature. This understanding proves vital in areas like biology, where researchers might track how certain environmental factors influence species populations, or in physics, where the interplay between forces is constantly being examined. In this article, we will explore what correlation data sets entail, their significance, and diving into methodologies and pitfalls.
So, letās unpack the pivotal findings surrounding correlation and how they shape scientific inquiry.
Foreword to Correlation Data Sets
Understanding correlation data sets lies at the heart of scientific research, underlining the intricate relationships among variables. It provides crucial insights, enabling scholars and practitioners to draw meaningful conclusions from their findings. Correlation, in its simplest terms, refers to a measure of the degree to which two variables move in relation to each other. This can manifest in various waysāpositively, negatively, or not at all.
In the realm of scientific inquiry, grappling with correlation can have profound implications. It informs hypotheses, guides experimental design, and influences data interpretation. For instance, recognizing a positive correlation between hours studied and test scores can help educators tailor their teaching methods or suggest effective study habits to students. Conversely, understanding negative correlations can shed light on potential causal relationships, urging researchers to delve deeper into the underlying dynamics.
Moreover, correlation data sets offer a framework for assessing the strength and significance of relationships in complex datasets. They are invaluable when exploring trends, especially across different fields such as psychology, economics, biology, or environmental science. As such, this article aims to demystify the concept of correlation for students, researchers, educators, and professionals, fostering a comprehensive understanding of its role in scientific research.
"Correlation does not imply causation, but it is a vital starting point for scientific exploration."
In the sections that follow, we will dissect the fundamental definitions, delve into the types of correlation, and explore the coefficients that quantify these relationships. The importance of these aspects cannot be overstated, as they lay the groundwork for robust data analysis. Through this exploration, readers will not only gain insights into correlation's theoretical framework but also practical tools to apply in their own research endeavors.
Defining Correlation
Correlation is often quantified by correlation coefficients, which offer a numerical value that indicates the strength and direction of a relationship between two variables. In clear terms, a positive correlation coefficient suggests that as one variable increases, the other tends to also increase. Conversely, a negative coefficient indicates that as one variable increases, the other decreases.
The correlation coefficient value ranges from -1 to +1. A value of +1 signifies a perfect positive correlation, -1 indicates a perfect negative correlation, and 0 reflects no correlation at all. These numeric values form the bedrock of data analysis in various disciplines.
Importance of Correlation in Research
Correlation plays a pivotal role in research for several reasons:
- Informative Insights: By analyzing correlations, researchers can uncover patterns that inform further inquiry.
- Hypothesis Testing: Correlation data serves as a foundation for constructing hypotheses, guiding studies aimed at understanding causative factors.
- Resource Optimization: Understanding relationships allows researchers to allocate resources more effectively, focusing on variables with significant correlations.
All these attributes combine to highlight the necessity of mastering correlation analysis, paving the way for deeper exploration and impactful findings in various branches of science.
Types of Correlation
Understanding the types of correlation is fundamental when exploring relationships between variables in research. Correlation indicates how one variable may change in relation to another, whether they move in tandem, the opposite direction, or not at all. Recognizing these distinctions can significantly affect the interpretation of study results and the outcomes of scientific inquiries. In this section, weāll dive into three primary types of correlation: positive, negative, and zero correlation. Each type sheds light on different patterns observed in data sets and helps us derive meaningful insights from our analyses.
Positive Correlation
A positive correlation indicates that as one variable increases, the other variable tends to increase as well. This kind of relationship is often depicted with a straight line on a graph, where both variables rise together. For instance, consider the relationship between hours spent studying and exam scores. Generally, the more time a student dedicates to studying, the higher their exam scores tend to be.
Key characteristics of positive correlation:
- Range: Values typically range between 0 and 1.
- Strength: The closer the correlation coefficient is to 1, the stronger the correlation. This can be a crucial factor in scientific research where precision matters.
"With a strong positive correlation, researchers can confidently predict outcomes based on input variables."
In practical applications, positive correlations are essential for making informed decisions in fields like education, marketing, and psychology. They can guide strategies for improving outcomes by focusing on enhancing the independent variable.
Negative Correlation
In contrast, negative correlation signifies that as one variable increases, the other decreases. This relationship can also be represented graphically, typically showing a downward slope. An example could be the relationship between the amount of time spent on social media and academic performance; as the time on social media increases, academic performance often declines.
Considerations regarding negative correlation:
- Indicating inverse relationships: It can reveal how various factors can counteract each other, providing insight into complex dynamics.
- Range: Values typically range from -1 to 0, where a value closer to -1 indicates a stronger negative correlation.
Researchers must tread carefully when interpreting negative correlations, considering contexts, as they might misinterpret causation or overlook other influencing variables.
Zero Correlation
Zero correlation is perhaps the most intriguing type because it implies no discernible relationship between the two variables. When graphed, the points appear scattered without a clear trend, suggesting that changes in one variable do not predict changes in the other at all.
A common example could be the relationship between a person's shoe size and their intelligence. No logical connection exists that suggests a correlation between these two variables.
Features of zero correlation include:
- Lack of predictability: Understanding that two variables do not influence each other is crucial for directing resources and efforts in research.
- Value range: Coefficients hover around 0, highlighting a complete independence between the two variables.
In various research contexts, identifying a zero correlation can be equally valuable as recognizing positive or negative correlations. It allows scientists to narrow down variables that influence outcomes and reject false assumptions in their studies.
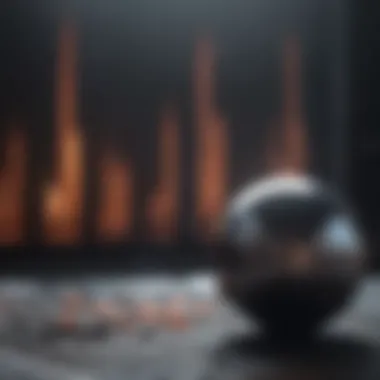
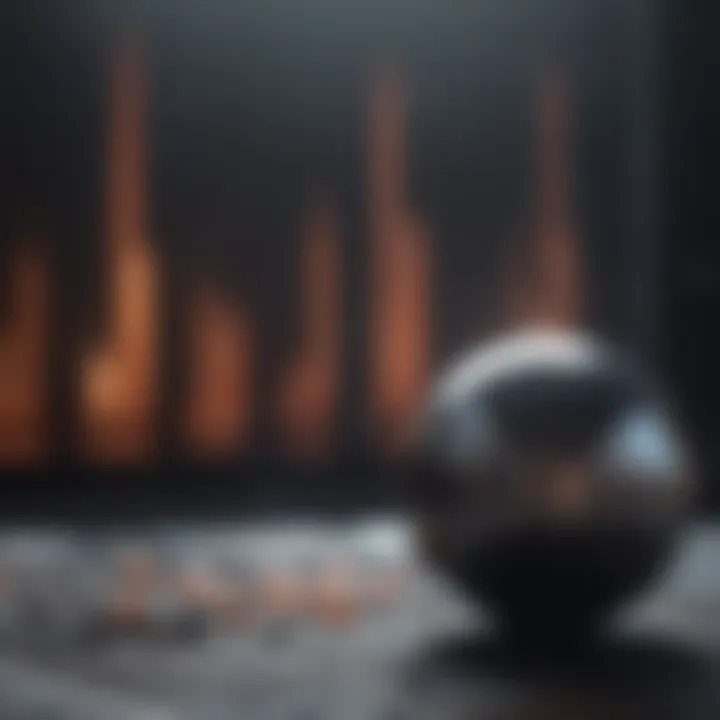
Correlation Coefficients Explained
Correlation coefficients serve as essential tools in the realm of statistical analysis, allowing researchers to quantify and interpret relationships between variables. The beauty of correlation coefficients lies in their ability to provide insights that can either validate or challenge hypotheses. Understanding these coefficients is crucial for anyone involved in scientific research, from students to seasoned professionals.
These coefficients are not mere numbers; they encapsulate the strength and direction of a relationship. Ranging from -1 to 1, they can signal a strong negative correlation (-1), no correlation (0), or a strong positive correlation (1). Grasping how to interpret these values not only informs decisions about future research but also aids in drawing meaningful conclusions from existing data.
Pearson Correlation Coefficient
The Pearson correlation coefficient, often denoted as "r," is one of the most recognized measures of correlation. This method is particularly useful when assessing linear relationships between two continuous variables. For instance, if a researcher wishes to explore whether there's a connection between study hours and exam scores, Pearson's r can provide clarity.
Pearson's coefficient calculation involves:
- Determining the covariance of the two variables, which measures how much they change together.
- Normalizing this value by the product of their standard deviations.
The result will indicate how closely the data points cluster around a line of best fit, effectively reflecting the linear nature of their relationship. However, it's vital to remember that Pearson's coefficient only captures linear relationships. If the data follows a non-linear pattern, relying solely on Pearson could mislead conclusions.
Spearman's Rank Correlation Coefficient
Spearman's rank correlation coefficient, represented as "Ļ" (rho), is a non-parametric measure that evaluates the strength and direction of association between two ranked variables. Unlike Pearson's method, Spearman does not assume a normal distribution and can thus handle ordinal data.
A classic example could involve ranking participants based on their performance in two different assessments. Spearman's coefficient assesses whether higher rankings in one variable correspond to higher rankings in another.
Calculating Spearman's coefficient involves:
- Ranking the data points within each variable.
- Finding the differences between ranks for each pair of scores.
- Applying the formula that incorporates these differences to yield a coefficient between -1 and 1.
This method is particularly advantageous when working with skewed data or when the relationship is not necessarily linear, giving researchers a more nuanced understanding.
Kendall's Tau Coefficient
Kendall's tau coefficient, denoted as "Ļ," is another non-parametric measure for assessing the strength and direction of association between two variables. Like Spearman's method, itās applicable for ordinal data but offers distinct advantages and approaches.
Kendallās tau evaluates the differences in ranks and is particularly noted for its robustness in small sample sizes. To calculate Kendall's tau:
- Identify the number of concordant and discordant pairs within the dataset.
- Apply the formula which uses these counts to yield a correlation coefficient.
Unlike Spearmanās, which essentially transforms the variables into ranks, Kendall's tau gives a clearer view of matched pairs, making it ideal for datasets where the ranks have inherent ties or for analysis with smaller datasets.
Ultimately, the choice of correlation coefficient should be dictated by the data's nature and the specific research questions at hand. Each coefficient offers unique insights and can help researchers navigate the complexities of correlation analysis with confidence.
Data Collection Methods for Correlation Studies
In the realm of scientific inquiry, the collection of reliable data holds paramount importance. For correlation studies, the methods employed to gather this data can significantly influence both the findings and their interpretation. After all, a sturdy framework built on robust data collection can support stronger conclusions and minimize false assumptions. Effective data collection not only lays the groundwork for solid analyses but also enables researchers to capture the nuanced relationships within their datasets.
When delving into correlation studies, one must consider how data is amassed, as this directly impacts the reliability of correlations drawn afterwards. Several techniques stand out, each with its own merits and demerits. Let's explore them in detail.
Surveys and Questionnaires
Surveys and questionnaires are often the bread and butter of data collection in correlation research. These tools allow researchers to gather a broad swath of information from a large number of participants, thus ensuring diversity and varied perspectives.
- Advantages:
- Cost-effective: They tend to be less expensive to administer, especially if distributed digitally.
- Time-efficient: Researchers can collect vast amounts of data relatively quickly.
- Anonymity: This can lead to more honest responses, especially on sensitive topics.
However, itās crucial to design these surveys thoughtfully. Questions must aim to eliminate bias and confusion. A poorly phrased question could skew results, resulting in misleading correlations. Hence, pilot testing the questionnaire is often recommended.
Experimental Design
Experimental design stands at the core of producing rigorous correlation data. It involves formulating a structured approach to manipulating one variable while observing the effects on another.
- Critical Considerations:
- Control Groups: Establishing a control group is necessary for minimizing outside influences that could confound results.
- Random Assignment: Ensures that differences between groups are due to the experimental treatment rather than pre-existing differences.
Using an experimental design means that while the focus is on eliciting correlation, causality can also begin to surface. For instance, if an experiment finds that increased sunlight correlates with greater plant growth, one can start to theorize about potential causal pathways or mechanisms involved.
Observational Studies
In situations where experimentation isnāt feasible or ethical, observational studies come into play. This method involves analyzing existing data or observing subjects in natural settings without any intervention.
- Pros:
- Real-world Applicability: These studies reflect actual conditions, offering insight into complex behaviors and relationships.
- Flexibility: Researchers can adapt their focus based on preliminary observations and emerging patterns.
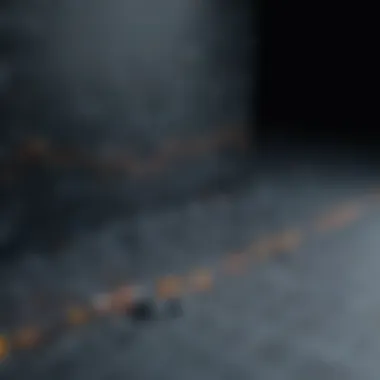
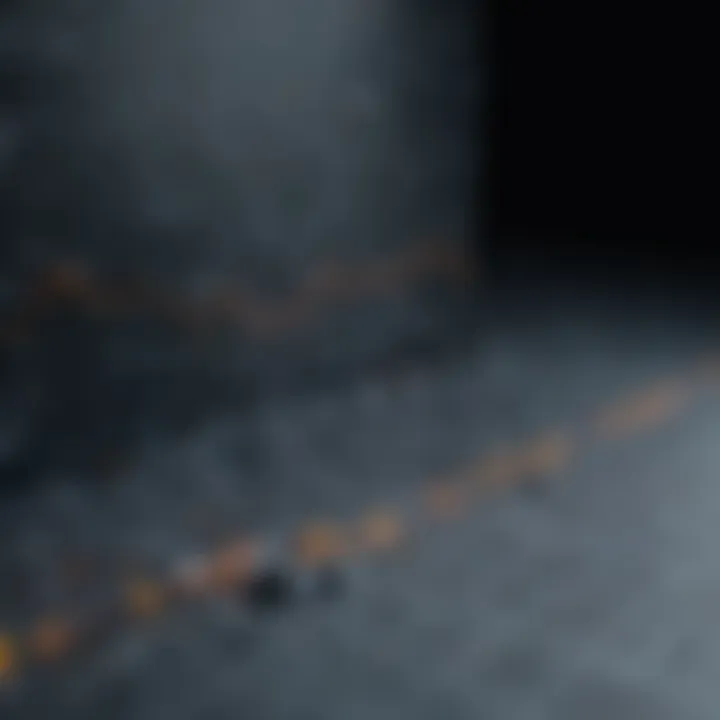
Nonetheless, observational studies carry a risk of confounding variables skewing the true nature of the relationships being studied. Careful control of potential extraneous variables is essential for gleaning meaningful correlations.
In summary, the method of data collection profoundly shapes the outcomes of correlation studies. Whether through surveys, experimental designs, or observational studies, each technique brings unique strengths and challenges that researchers must navigate with precision.
Data Analysis Techniques
In any piece of research, particularly in the realm of correlation studies, how you analyze your data stands as a pillar of your findings. The ability to extract actionable insights from raw numbers not only enhances the credibility of your work but also paves the way for informed decision-making in scientific practices. Through these techniques, researchers can decipher complex relationships between variables, uncover hidden patterns, and interpret results in a way that contributes to broader conversations in their fields.
Statistical Software Utilization
Statistical software has become a staple in the arsenal of researchers embarking on correlation analysis. Tools such as R, SPSS, and Python libraries like Pandas and NumPy provide an immense capability to handle data-intensive tasks. Utilization of these programs offers several advantages:
- Efficiency: Large datasets can be daunting and time-consuming to analyze manually. Statistical software streamlines this process, allowing for quick computation of correlation coefficients and other statistical measures.
- Accuracy: With built-in functions, these tools reduce human error that can easily creep in during manual calculations.
- Advanced Analysis: Beyond simple correlation calculations, these software packages allow deeper dives into regression analysis, multivariate studies, and more, expanding the scope of analysis.
However, while these tools are incredibly powerful, itās crucial for users to have a basic understanding of statistical principles. Misinterpretation of outputs can lead to catastrophic conclusions. A researcher using SPSS in a healthcare study, for instance, might find a strong positive correlation between exercise and well-being, but without understanding the underlying statistical principles, they risk drawing misguided inferences about causation.
Visual Representation of Correlations
Seeing data visually can often bring clarity where numbers alone may confound. Visual tools like scatter plots, heat maps, and correlation matrices provide an intuitive grasp of relationships between variables.
- Scatter Plots: These are particularly effective in illustrating the relationship between two variables, making it easy to see trends and exceptions. A well-placed scatter plot might display the connection between temperature and ice cream sales, allowing for immediate visual interpretation.
- Heat Maps: For datasets with multiple variables, heat maps can quickly indicate where strong correlations exist and where they do not. When analyzing student performance across various subjects, a heat map might visually represent the correlation between mathematics and science scores, highlighting areas of strength or need for intervention.
- Correlation Matrices: These succinctly provide a comprehensive overview of how multiple variables interact with one another, offering a quick reference for researchers looking to understand their data landscape at a glance.
Using visual aids not only facilitates better comprehension but also becomes an important communication tool when sharing findings with a broader audience who may not have a statistical background. As they say, āA pictureās worth a thousand words.ā
Interpreting Correlation Outputs
Analyzing and interpreting correlation data can often feel like putting together a puzzle. Each piece must be carefully examined and positioned to understand the full picture. Here are several considerations researchers should keep in mind while making sense of their statistical outputs:
- Significance Levels: Understanding the p-value alongside correlation coefficients is critical. A low p-value (typically 0.05) indicates that the observed correlation is statistically significant, reducing the likelihood that it occurred by chance.
- Strength of Correlation: The coefficient value itself indicates the strength and direction of the relationship. A value closer to 1 or -1 signifies a strong correlation, while values near 0 indicate weak correlation signals.
- Contextual Clarity: Output should be interpreted within the context of the studyās design, methodology, and surrounding literature. For example, a strong negative correlation between hours of sleep and stress levels across a sample of graduate students may make sense in light of common academic pressures.
- Causation vs. Correlation: Regardless of how strong a correlation appears, itās crucial to remember that correlation does not imply causation. For instance, while consuming more ice cream might correlate with high rates of drowning, the underlying common factor is likely summertime rather than a direct relationship between the two.
Case Studies: Applications of Correlation Data Sets
The realm of correlation data sets finds its true significance through practical applications, which are well illustrated in various fields of scientific inquiry. Case studies provide concrete examples where correlation analysis has not only elucidated relationships between variables but also paved the way for new insights and knowledge. The decision to utilize case studies amplifies understanding by demonstrating tangible implications of theoretical concepts. Each example stands as a testament to the power of correlation in drawing connections between seemingly disparate data points and often leads to broader conclusions that can impact future research.
Correlation in Biology
In the field of biology, correlation data sets can be seen as the backbone of understanding relationships within ecosystems, genetic traits, or disease patterns. For instance, researchers may explore the relationship between the consumption of certain nutrients and the incidence of diseases like diabetes. A notable study published in the New England Journal of Medicine examined dietary habits and found significant correlations indicating that higher intake of whole grains was associated with lower rates of type 2 diabetes.
This kind of analysis not only sheds light on potential health interventions but also helps in understanding broader biological phenomena such as overall human health and nutrition. Furthermore, observing the correlation between environmental factors and species population dynamics informs conservation efforts. If a biologist finds a strong correlation between higher temperatures and reduced fish populations in a particular habitat, this could drive policy changes to address climate impacts.
Correlation in Physics
Physics, often seen as a realm governed by clear laws and rigid formulas, also utilizes correlation analysis to strengthen its theories and predict outcomes. Take, for example, the correlation between the speed of an object and the distance it travels over time. Through numerous experiments, physicists have gathered data that shows a predictable relationship under constant acceleration.
A classic case can be illustrated with the study of projectile motion. By analyzing the correlation between angle of launch and distance traveled, researchers can derive equations that help predict trajectories. This correlation becomes particularly vital when developing technologies such as missile guidance systems or sports equipment like golf balls, where understanding the factors affecting distance traveled can optimize performance.
Correlation in Earth Sciences
Earth sciences heavily rely on correlation data sets to track patterns and changes over time. For example, researchers investigating climate change often analyze the correlation between carbon dioxide levels and global temperature rises. Studies by agencies like NASA have confirmed a strong positive correlation, indicating that as carbon dioxide levels rise, global temperatures tend to increase too.
This correlation underscores the urgency of understanding climate dynamics and serves as a call to action for policymakers. Additionally, geomorphologists may explore the correlation between rainfall patterns and soil erosion in specific regions. By illuminating these relationships, they can recommend appropriate land management practices to mitigate erosion, especially in vulnerable areas.
"Understanding the interplay of variables in natural phenomena transforms our approach to environmental conservation and disaster prevention."
In each of these areasābiology, physics, and earth sciencesācase studies showcase how correlation data sets are not mere academic exercises but vital tools that facilitate real-world decisions and innovations. By drawing on these examples, research can evolve, shaping scientific discourse and contributing to a deeper comprehension of complex systems.
Limitations of Correlation Data
Understanding the limitations of correlation data is pivotal in discerning how relationships between variables can be misinterpreted. Correlation studies certainly shine a light on potential connections but come with a few caveats that researchers must address thoughtfully.
Causation vs. Correlation
A common stumbling block in correlation research is the tendency to conflate correlation with causation. Just because two variables change in tandem doesnāt mean one causes the other. Consider a classic example: ice cream sales and drowning incidents. As temperatures rise in summer, more ice cream is sold, and at the same time, the number of drowning cases may increase. Does this imply that eating ice cream leads to drowning? Absolutely not. Itās simply a case of coincidental patterns due to a third variable ā the heat of summer.
A deeper look at this relationship reveals the danger of drawing conclusions from correlation alone. Researchers need to employ methodologies such as controlled experiments to establish causative influence genuinely. Misinterpretation can lead to flawed policies or misguided interventions that may not address the root cause of an issue. This underscores the importance of adopting a critical mindset when analyzing correlation data.
Sample Size and Its Impact
Another significant limitation pertains to sample size. A common maxim in research is that size matters. A small sample might not represent the population adequately, leading to skewed results. Suppose a study investigates the correlation between exercise frequency and weight loss using just a handful of participants. The findings may show a strong correlation, but how reliable is that conclusion when applied to a broader population?
On the flip side, while larger sample sizes can enhance the reliability of correlation findings, they also bring their challenges. For instance, if researchers collect an excessive amount of data without proper categorization or filters, it might create noise rather than clarity. As such, more data doesnāt always mean better understanding.
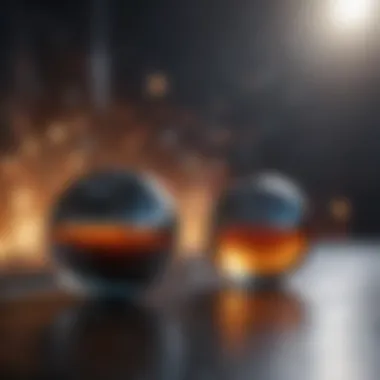
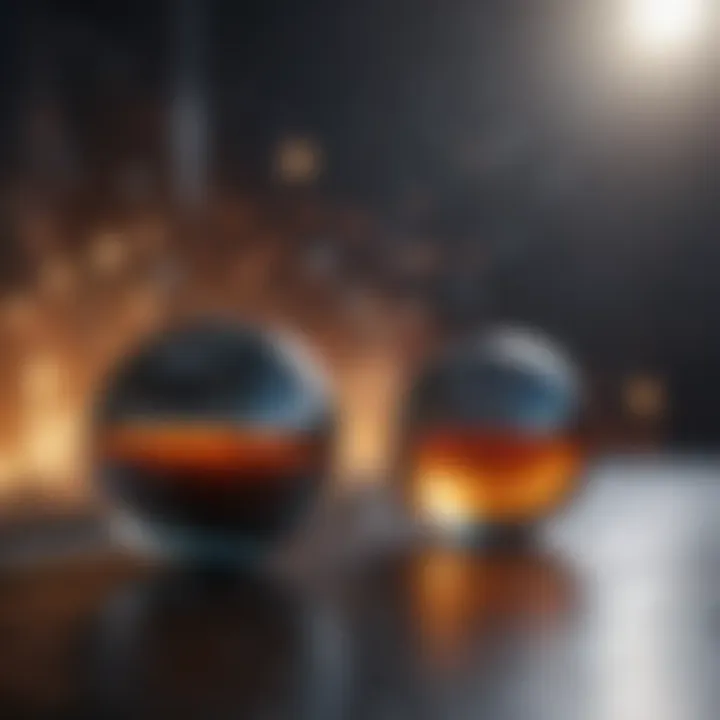
In practical terms, determining an appropriately sized sample involves balancing statistical power and practical constraints like available resources and time. Researchers must ask themselves:
- Is the sample large enough to capture the variability in the data?
- Are there enough participants to achieve statistically significant results?
Ultimately, while correlations offer valuable insights into relationships, a prudent approach to limitations like causation confusion and the requirements of a robust sample size is essential for genuine understanding in scientific inquiry.
"The temptation to draw conclusions from correlation without thorough investigation can lead even the most seasoned researchers astray."
By recognizing these limitations and accounting for them, researchers and practitioners can better utilize correlation data to advance their fields, ensuring that insights gained contribute to meaningful outcomes.
Ethical Considerations in Correlation Research
Ethics in correlation research plays a crucial role, significantly impacting the integrity and applicability of findings. To ensure that research meets ethical standards, researchers must address various factors when designing and conducting studies. These considerations involve safeguarding participant rights and fostering a trustworthy environment for data collection and analysis.
Informed Consent
Informed consent stands as a cornerstone of ethical research practice. It refers to the process wherein participants are fully informed about the study's purpose, procedures, risks, and benefits before agreeing to partake. This process embodies respect for individual autonomy and empowers participants to make educated decisions regarding their involvement.
An effective informed consent process typically includes the following steps:
- Clear Communication: Use straightforward language to articulate the studyās aims. Avoid jargon that might confuse participants.
- Time for Questions: Allow participants ample time to ask questions or seek clarification about what their involvement entails.
- Voluntary Participation: Emphasize that participation is purely voluntary and that participants can withdraw at any point without any negative repercussions.
- Documentation: Obtain signed consent forms from participants, ensuring they comprehend what they are consenting to.
Implementing these practices not only enhances ethical standards but also strengthens the validity of the research. Participants who understand the study are more likely to provide genuine responses, contributing to more reliable correlation data.
Data Privacy
Another vital aspect of ethical considerations in correlation research is data privacy. Researchers must prioritize the confidentiality and security of participants' information throughout the research process. Violating data privacy can lead to severe trust issues and discourage participant involvement in future studies.
Some essential practices for safeguarding data privacy include:
- Anonymization: Remove any identifying information from the data set to prevent tracing back results to individual participants.
- Secure Data Storage: Utilize encrypted platforms to store data, thereby minimizing unauthorized access or data breaches.
- Access Control: Limit access to sensitive data to only essential personnel. Establish protocols for how data can be shared and with whom.
- Informed Deletion Policies: Inform participants about how long their data will be retained and provide options for data deletion after the study is completed.
Practicing robust data privacy measures not only fulfills ethical obligations but also enhances the credibility of the research. Participants are more inclined to engage if they feel confident that their information is handled responsibly.
Ethical considerations are not just formalities, but integral components that shape the foundation of credible and reliable research.
In summary, addressing ethical considerations in correlation research is fundamentally about respecting participants and ensuring that data collection and analysis are conducted with integrity. By focusing on informed consent and data privacy, researchers bolster the moral framework of their work, ultimately contributing to the field's credibility and the useful application of research findings.
Future Directions in Correlation Research
As we step into an era saturated with data, the importance of exploring future directions in correlation research unfolds like a well-worn map. This section clarifies key areas of potential advancements, emphasizing not just the tools at our disposal but also the interdisciplinary connections that can elevate our understanding of data relationships. Given the rapid pace of technological evolution and the increasing complexity of scientific questions, this investigation reveals much about the next frontier in correlation research.
Emerging Technologies
Emerging technologies are shaping the landscape of correlation research, akin to a high-speed train weaving through the heart of unexplored territories. With the advent of machine learning and artificial intelligence, researchers can now analyze vast amounts of data in ways that were previously infeasible. Automated systems can reveal patterns that human analysts might overlook, allowing for the identification of nuanced correlations among variables.
Some notable technologies that are at the forefront include:
- Machine Learning Algorithms: Tools like random forests and neural networks facilitate more robust analyses by learning from data patterns without explicit programming. This empowers researchers to predict outcomes based on correlation findings more efficiently.
- Big Data Analytics: Platforms capable of processing enormous data sets provide a fertile ground for discovering new relevant correlations, especially in fields like genomics and environmental science.
- Cloud Computing: This increases accessibility and collaboration, enabling researchers across the globe to share and analyze data in real-time, breaking down previously thought barriers to data use.
These technologies do not just add granularity; they also enhance the potential for more profound insights, hurrying up the pace at which research progresses.
Interdisciplinary Approaches
The future of correlation research is not only about the technology but also about how diverse fields intersect with one anotherāmuch like how rivers branch into a wide delta. Cross-disciplinary collaboration is becoming increasingly vital as researchers look to narrow down on complex issues that no single discipline can tackle alone. For instance, the merging of biology, computer science, and social sciences can elucidate correlations affecting public health, as changing environmental factors interplay with social variables.
Important considerations for fostering interdisciplinary approaches include:
- Collaborative Research: Engaging experts from complementary fields encourages innovative methodologies and fresh perspectives that can reveal novel correlations.
- Shared Frameworks: Developing cohesive frameworks allows for communicative clarity between different scientific vocabularies. This helps in making complex correlation findings understandable across disciplines.
- Funding Opportunities: An increase in funding aimed specifically at interdisciplinary projects could accelerate breakthroughs in correlation research. Multiple disciplines joining forces often attract attention from funding bodies eager to promote holistic scientific investigation.
Understanding the connections between various fields can illuminate paths that might otherwise remain obscured, paving the way for groundbreaking findings and a richer comprehension of data relationships.
By embracing emerging technologies and interdisciplinary approaches, correlation research stands not just to thrive but to evolve into a more nuanced and powerful tool within the scientific toolkit. As the landscape shifts, it remains crucial for researchers to remain agile and curious, exploring uncharted waters in data relationships and correlations.
Ending
To wrap up our exploration into correlation data sets, itās crucial to emphasize the significance of the conclusion within this article. Understanding correlation isnāt just a statistical exercise; itās a lens through which we can view relationships and patterns across multiple scientific fields. In our increasingly data-driven world, the ability to effectively analyze and interpret these correlations can lead to groundbreaking discoveries or even significant advancements in various disciplines.
Summarizing Key Insights
As weāve journeyed through the intricacies of correlation, several key insights have emerged:
- Correlation Types: Weāve delved into positive, negative, and zero correlations, each presenting unique insights into data relationships. Recognizing these types is essential in research, as they set the stage for further analysis.
- Correlation Coefficients: The examination of Pearson, Spearman, and Kendall's Tau coefficients highlights the diverse methods available for quantifying relationships. Each coefficient provides a different perspective, tailored for specific types of data.
- Data Collection and Analysis: Understanding how to gather data through surveys, experiments, or observational studies builds a strong foundation for research. This collection process is vital, as it directly influences the results and conclusions drawn from a study.
- Limitations and Ethical considerations: Throughout our discussion, acknowledging the limits of correlation is paramount. Correlation does not imply causation, and researchers must remain vigilant about sample size and ethical considerations to ensure that their findings are not only valid but also morally sound.
The Importance of Critical Evaluation
Critical evaluation of correlation data is non-negotiable in scientific research. No study is immune to bias or misinterpretation, so examining findings from various angles can safeguard against flawed conclusions. Students and researchers must rigorously assess whether observed relationships hold up against scrutiny, and itās vital to cultivate a mindset that questions the validity of correlation analyses. Discerning readers should ask themselves:
- What is the source of this data?
- Are alternative interpretations of the data considered?
- Have the implications of the findings been explored thoroughly?