Understanding the Forecasting Equation: Principles and Applications
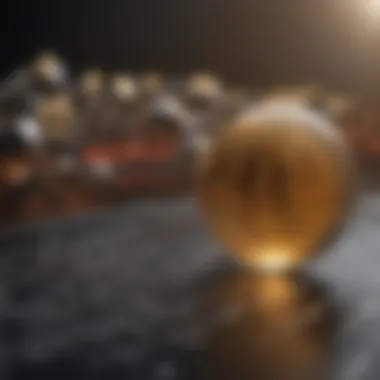
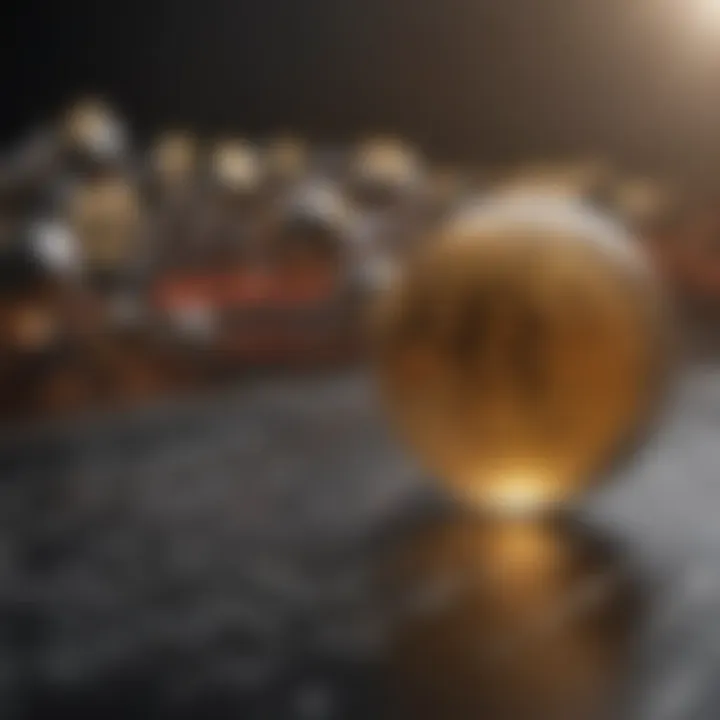
Intro
Forecasting is a fundamental aspect of scientific inquiry and decision-making. It enables researchers, educators, and professionals to anticipate future events based on historical data. However, to effectively utilize this forecasting capability, one must understand the underlying principles and mathematical foundations of the forecasting equation. This article will explore these complexities, providing practical insights and strategies that enhance predictive accuracy in various scientific disciplines.
The forecasting equation itself serves as a powerful tool, meant to translate past observations into future predictions. It encompasses various methods, each with its own strengths and weaknesses. Throughout this discussion, we aim to clarify key concepts while identifying real-world applications that encompass diverse fields, from economics to environmental science.
In addition, we highlight common challenges faced in forecasting. Understanding these issues is essential for improving model reliability and interpretation. Through this exploration, we will illuminate the significant role that the forecasting equation plays in advancing research and practice across disciplines.
Key Findings
Summary of the Main Results
This section will outline several critical findings related to the forecasting equation:
- Mathematical Foundations: The equation relies on a set of mathematical principles including regression analysis, time series analysis, and statistical inference. Each component contributes uniquely to enhancing the accuracy of predictions.
- Practical Applications: The forecasting equation is adaptable to a range of fields. For instance, in economics, it predicts market trends, while in environmental science, it forecasts climate change impacts.
- Common Challenges: Various issues arise in the application of forecasting methods. These include data quality, model selection, and interpretation of results. Each challenge presents an opportunity for ongoing research and refinement of techniques.
Significance of Findings Within the Scientific Community
The significance of these findings cannot be overstated. As research becomes increasingly data-driven, the forecasting equation emerges as a critical tool integral to scientific exploration. Embedding these principles into practice fosters a more robust understanding of phenomena. Furthermore, the potential to refine forecasting models promises substantial advancements in predictive science.
"An effective forecasting equation can bridge the gap between research findings and practical implementation, allowing for informed decision-making across sectors."
Implications of the Research
Applications of Findings in Real-World Scenarios
The insights gained from understanding the forecasting equation are applicable in numerous real-world scenarios:
- Business Planning: Companies utilize these principles to project sales, manage inventory, and optimize marketing strategies.
- Public Policy: Governments can apply forecasting models to identify social trends, allocate resources, and develop effective policies.
- Healthcare: The forecasting equation assists in predicting disease outbreaks and managing patient loads.
Potential Impact on Future Research Directions
The implications of the research extend beyond immediate applications. A deeper understanding of forecasting can inspire new methodologies and interdisciplinary collaboration. It encourages researchers to refine models and explore innovative solutions to complex problems. The continual evolution of forecasting practices holds the potential to enhance academic and practical outcomes significantly. By integrating advanced techniques, future research can better address uncertainties and improve overall predictive capabilities.
Prolusion to Forecasting Equations
Forecasting equations play a crucial role in the realm of predictive analytics across a wide array of scientific disciplines. Understanding these equations is vital for accurately predicting future events based on historical data. Various fields, including economics, biology, and meteorology, utilize these equations to make informed decisions by interpreting trends and patterns found in past information.
In this article, we will delve into the foundational principles that govern forecasting equations. By doing so, we aim to provide insights into their construction, the reasoning behind their applications, and potential advantages. The importance of mastering forecasting equations extends beyond mere academic interest; it is essential for researchers and practitioners who seek to refine their analytical capabilities.
Definition and Importance
Forecasting can be defined as the process of estimating future outcomes based on historical data. This concept is grounded in the idea that past trends often provide essential context for predicting future events. The importance of this lies in its practical applications.
- Informed Decision-Making: Business leaders rely on forecasting models to strategize and minimize risks.
- Resource Allocation: Effective forecasting can help in distributing resources where they are most needed.
- Trend Analysis: Academics and researchers can identify significant patterns that lead to new discoveries.
Thus, grasping the definition and importance of forecasting equations paves the way for deeper comprehension of their operational mechanics and applications.
Historical Context
The history of forecasting dates back centuries, with early examples found in agricultural predictions and astronomical observations. Methods and tools have evolved significantly over time.
- Early Tools: Ancient civilizations used rudimentary observations and seasonal changes to forecast agricultural yields.
- Advancements in Mathematics: The 20th century saw significant advancements in statistical techniques, which allowed for more rigorous and reliable forecasting methods. Tools like linear regression emerged around this time.
- Data-Driven Era: The advent of computer technology in the late 20th century has transformed forecasting practices. Modern techniques leverage vast data sets and sophisticated algorithms to generate accurate predictions.
In summary, understanding the historical context of forecasting enhances oneβs appreciation for its complexity and importance in modern scientific research.
Theoretical Framework of Forecasting
The theoretical framework of forecasting is crucial for understanding how predictions are made in various fields. This framework helps establish a systematic approach to analyzing data, predicting future trends, and making informed decisions based on insights gathered from historical information. The importance lies in its ability to structure the forecasting process and provide clear methodologies that can be applied across different disciplines.
Types of Forecasting
Qualitative Forecasting
Qualitative forecasting refers to methods that rely heavily on expert opinions and subjective judgments. This approach is particularly useful when historical data is scarce or non-existent. One significant aspect of qualitative forecasting is its flexibility in adapting to changing circumstances. Experts can incorporate new insights or shifting trends that quantitative models may overlook.
The key characteristic of qualitative forecasting is its emphasis on human judgment, making it a beneficial choice for areas such as market research or new product development. A unique feature of this method is its reliant on the experience and intuition of individuals, allowing for the incorporation of insights that statistical methods might miss. However, it is essential to note that subjective opinions can introduce bias, which can affect accuracy.
Quantitative Forecasting
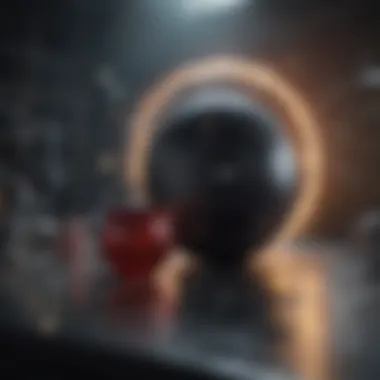
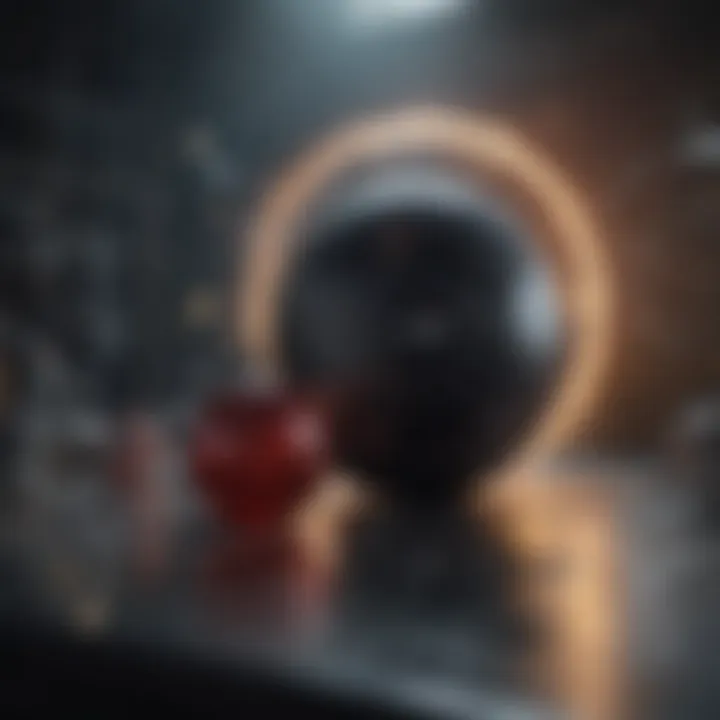
Quantitative forecasting, on the other hand, involves systematic data analysis using statistical techniques. It is primarily used when historical data is available and offers a more objective means of making predictions. The strength of quantitative forecasting lies in its ability to provide measurable insights that can be statistically validated.
The key characteristic of quantitative forecasting is its reliance on numerical data, making it popular in fields such as finance and supply chain management. The unique feature of quantitative methods is their ability to process large datasets and identify trends or patterns that may not be immediately apparent. However, one downside to quantitative forecasting is its dependency on historical data, meaning it may be less effective in unpredictable environments.
Statistical Principles
Probability Theory
Probability theory is a fundamental component of forecasting that deals with uncertainty. It provides a structured means of quantifying the likelihood of various outcomes. This aspect is particularly important in forecasting since predictions inherently involve uncertainty.
The key characteristic of probability theory is its focus on modeling and quantifying uncertainty, making it a beneficial tool in many forecasting scenarios. A unique feature is that it allows forecasters to express predictions in probabilistic terms, providing a clearer picture of potential scenarios. However, its complexity can sometimes lead to misunderstandings if the underlying concepts are not well grasped.
Regression Analysis
Regression analysis plays a crucial role in forecasting, as it enables the exploration of relationships between variables. By identifying how different factors influence each other, forecasters can create models that predict future values based on historical data. The key characteristic of regression analysis is its ability to formulate equations that capture these relationships, making it incredibly valuable in quantitative forecasting.
The unique feature of regression analysis is its versatility in handling multiple variables, which can enhance predictive accuracy. Despite its advantages, it also has limitations, such as the potential for overfitting when models become too complex, leading to inaccurate predictions in new instances.
Mathematical Foundations of the Forecasting Equation
The mathematical foundations of the forecasting equation are critical for building reliable predictive models. They provide the underlying structure that allows researchers to quantify relationships between different factors. Understanding these foundations enables practitioners to make informed decisions based on accurate predictions. Mastery of the mathematical elements involved in forecasting allows for better analysis of various scientific phenomena. It aids in translating real-world processes into comprehensible mathematical terms, which is key to any forecasting endeavor.
Basic Components
Dependent Variables
Dependent variables represent the outcomes that forecasting models aim to predict. Understanding these variables is essential, as they are the focal point of any predictive analysis. The key characteristic of dependent variables is that they are influenced by independent variables.
For example, in an economic forecasting model, the dependent variable could be GDP growth, which is influenced by many factors like interest rates and inflation. This makes the dependent variable a beneficial choice, as it ties directly to the end goal of any forecasting effort.
However, a unique feature of dependent variables is their sensitivity to change. External factors can dramatically influence predictions, making it a double-edged sword. On the one hand, its predictive power is useful; on the other hand, it can lead to variability in accuracy, depending on how well other variables are understood.
Independent Variables
Independent variables are the factors that influence the dependent variable. Their importance lies in their ability to provide explanations for changes in the dependent variable. The key characteristic of independent variables is that they are not affected by other variables in the equation, making them a crucial component in the modeling process.
For instance, in predicting sales revenue, independent variables could include advertising expenditure and market conditions. The benefit of using independent variables is their capacity to help researchers isolate the specific factors that drive changes in the dependent variables.
Their unique feature is that they can be selected based on theoretical considerations or empirical analysis. This adaptability aids researchers in developing more tailored forecasting models. However, the challenge lies in correctly identifying relevant independent variables, as the wrong choice can lead to poor predictions and reduced model reliability.
Formulating the Equation
Linear Models
Linear models serve as a fundamental approach in forecasting due to their simplicity and ease of interpretation. They utilize a straight-line representation to relate independent variables to the dependent variable. The main advantage of linear models is that they allow for straightforward calculations and clear insights into the relationships among variables.
The key characteristic that makes linear models a popular choice is their ability to handle multiple inputs efficiently. This characteristic allows for quick assessments and rapid predictions. They are suitable when relationships among variables are assumed to be constant, which simplifies the overall analysis.
However, a downside of linear models is their limitations in capturing complex relationships. Many real-world scenarios involve nonlinear interactions, which linear models may not accommodate adequately, potentially leading to biased estimates.
Nonlinear Models
Nonlinear models offer a contrasting approach by allowing for a more flexible relationship between independent and dependent variables. This flexibility is particularly useful in scenarios where relationships are not strictly linear. A notable feature of nonlinear models is their ability to address real-world complexities more effectively than linear models.
Nonlinear models are beneficial if there are indications that the relationship between variables changes at different levels. For example, in ecological forecasting, population growth can exhibit exponential qualities under certain conditions, which linear models might fail to capture. This ability to adapt to the intricacies of data is a significant advantage of nonlinear models.
However, one of the challenges with nonlinear models is their complexity. They often require intricate estimation techniques, making them more demanding in terms of computation and analysis than linear models. This complexity can lead to difficulties in interpretation, requiring careful consideration when applying these models in practical scenarios.
Techniques for Developing Forecasting Models
Developing forecasting models is critical for effective decision-making in various fields. The techniques used in forecasting models can significantly impact the accuracy of predictions based on historical data. Selecting the right method can lead to more reliable outcomes, while poor choices might result in misleading insights. Building a robust model involves a nuanced understanding of both the data used and the methodologies applied.
Data Collection and Preparation
Sources of Data
In forecasting, the initial data is fundamental. Sources of data can vary widely and include academic research, industry reports, market surveys, and public databases. Each source has its own set of unique characteristics. For example, academic research often provides peer-reviewed data, which can enhance reliability. However, it may not always reflect real-time changes in the industry.
Utilizing industry reports might deliver up-to-date market information, but these can sometimes be biased or selectively presented. Combining multiple sources typically enhances data diversity, allowing for a more holistic view. This approach ensures that the forecasting models created are grounded in comprehensive datasets, increasing their effectiveness in analyzing potential future outcomes.
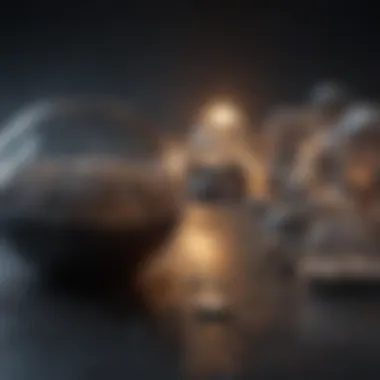
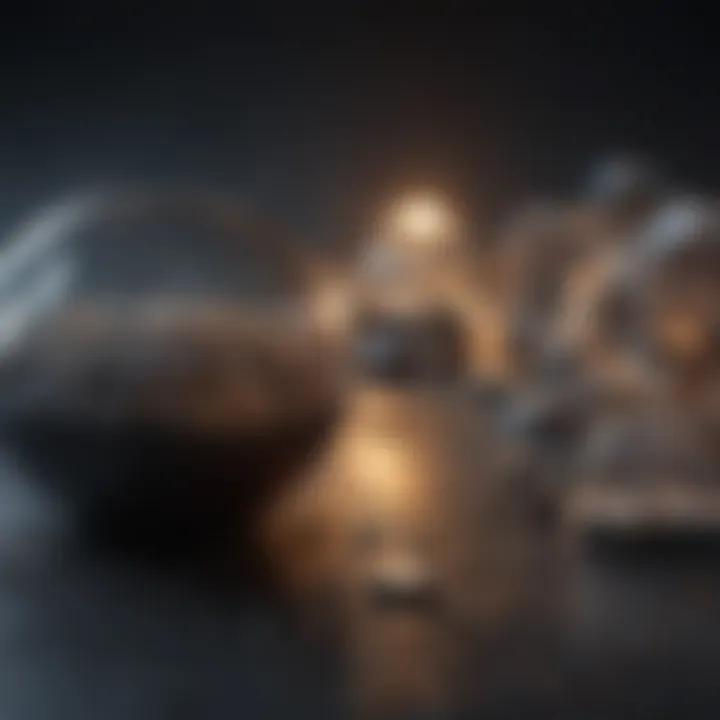
Data Cleaning Procedures
After sourcing data, the next crucial step is data cleaning. This process entails identifying and correcting inaccuracies, inconsistencies, or incomplete information within datasets. Data cleaning is not just beneficial; it is essential. A model built on flawed data can lead to faulty predictions.
Key characteristics of effective data cleaning procedures include the removal of duplicates, handling missing values, and standardizing formats. Each of these practices contributes to a dataset that is not only cleaner but also more streamlined for analysis. While data cleaning can be time-consuming, its unique feature lies in significantly reducing the risk of errors that could compromise the integrity of predictions.
Model Selection and Validation
Training and Testing Data
When developing forecasting models, splitting data into training and testing sets is a common practice. Training data is used to build the model, while testing data assesses the model's accuracy. This process ensures that the model generalizes well to unseen data.
One of the key characteristics of this approach is its ability to help identify overfitting. Overfitting occurs when a model performs well on training data but fails miserably on new data. Regularly splitting the dataset into distinct training and testing portions allow researchers to calibrate their models systematically, improving overall reliability.
Cross-Validation Techniques
Cross-validation techniques further enhance the validation of forecasting models. Instead of a simple train/test split, these methods involve dividing the dataset into multiple subsets. The model is then trained and validated several times, rotating the data used for training and testing in each iteration. This distinct characteristic allows for a more thorough assessment of the model's performance.
The unique feature of cross-validation is its focus on model robustness. By employing this technique, forecasters can mitigate biases that may arise from a single train/test split. However, it is essential to acknowledge that this method requires more computational resources and time than simple validation methods, which can be a potential disadvantage in scenarios with limited resources.
"Effective forecasting is a blend of rigorous data preparation and a solid model selection process."
Through these techniques, stakeholders are better positioned to obtain accurate predictions tailored to their specific fields, whether that be biology, economics, or meteorology. Employing solid data practices and validation techniques can not only improve forecasting models but also facilitate sound interpretations of results.
Applications Across Scientific Disciplines
The application of forecasting equations extends significantly across various scientific disciplines. Understanding these applications is crucial for comprehending how theoretical concepts translate into practical outcomes. Each field approaches forecasting in unique ways, offering insights and methods that facilitate the prediction of future trends and behaviors.
The integration of forecasting in distinct scientific areas enhances accuracy and relevance. It allows researchers, educators, and practitioners to utilize these tools effectively. Different disciplines share general principles but adapt methodologies according to their specific needs. This adaptability is a noteworthy consideration in forecasting practices.
Forecasting in Biology
Population Dynamics
Population dynamics is central to biological forecasting. This field studies the changes in population sizes and composition over time. It significantly contributes to understanding ecological balance, species interactions, and resource management. The crucial aspect of population dynamics is its ability to model complex interactions within ecosystems.
One key characteristic of population dynamics is its focus on birth rates, death rates, and migration patterns. This characteristic makes it a beneficial choice for researchers studying species survival and ecological stability. A unique feature of this approach includes the use of mathematical models that represent interactions within populations. The advantages lie in its ability to predict long-term trends in species abundances, while disadvantages include the challenges of data availability and accuracy.
Ecological Modeling
Ecological modeling serves as another essential tool. It encompasses simulating ecosystems to assess their behavior under various scenarios. This contribution is vital for the understanding of environmental changes and biodiversity. The key characteristic of ecological modeling is its holistic approach, integrating multiple variables that influence ecological outcomes.
This method's popularity stems from its effectiveness in predicting responses to environmental changes, thus allowing for informed decision-making in conservation. A unique feature is its capacity to assess system resilience and sustainability. However, a disadvantage includes its potential over-simplification of complex natural processes, which can lead to misleading conclusions.
Forecasting in Economics
Market Trends Analysis
Market trends analysis focuses on the economic landscape, providing insights into consumer behavior and market fluctuations. Its contribution lies in helping businesses and investors to make data-driven decisions. The crucial aspect of this analysis is its reliance on historical data to forecast future trends.
Market trends analysis is favored for its ability to gauge economic conditions quickly. Its unique feature involves analyzing various indicators like consumer spending, inflation, and employment rates. The advantage of this method is its potential to predict economic downturns or booms. However, it can face limitations due to the unpredictability of external factors influencing market dynamics.
Financial Predictions
Financial predictions are specifically directed towards future performance of assets or markets. This aspect is fundamental for investors and financial analysts. Its contribution to economic forecasting is immense, as it can inform strategies and risk assessments. A key characteristic is its use of quantitative techniques to project outcomes.
Financial predictions are particularly beneficial in volatile markets where swift adjustments are necessary. The method's unique feature is its combination of statistical techniques and market analysis. Advantages include identifying lucrative investment opportunities, while disadvantages revolve around the reliance on historical data, which may not always predict future trends accurately.
Forecasting in Meteorology
Weather Prediction Models
Weather prediction models play a pivotal role in forecasting atmospheric conditions. This aspect is crucial for public safety and preparedness. The contribution of weather models lies in their ability to deliver timely warnings about severe weather conditions. A key characteristic of these models is their reliance on vast amounts of data collected from various sources.
These models are beneficial due to their sophisticated algorithms that analyze complex atmospheric behaviors. A unique feature is the integration of satellite data and ground observations, enhancing predictions' accuracy. However, disadvantages include the unpredictability of weather patterns, leading to occasional inaccuracies in forecasts.
Climate Change Projections
Climate change projections are critical for understanding long-term environmental changes. This aspect allows scientists to assess the potential impacts of climate change. Its contribution is indispensable in informing policy and conservation efforts. The key characteristic is its reliance on computer models that simulate future climate scenarios.
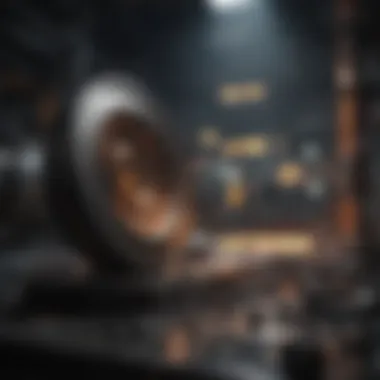
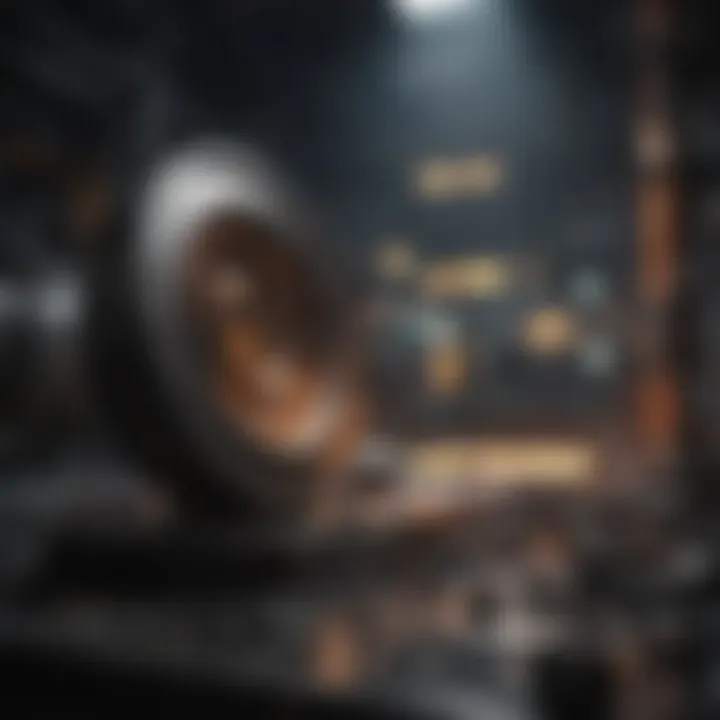
These projections are essential for guiding global efforts to mitigate climate impacts. A unique feature is the incorporation of various greenhouse gas emission scenarios and their effects. Advantages include raising awareness and urging action, whereas disadvantages pertain to uncertainties inherent in modeling long-term climate trends.
Challenges in Forecasting Methods
Understanding the challenges in forecasting methods is crucial when working with the forecasting equation. The predictive process is influenced by numerous factors that can distort outcomes or make them unreliable. Addressing these challenges early on can significantly contribute to enhancing predictive accuracy. This section will explore two major aspects: dealing with uncertainty and the issues of overfitting and underfitting.
Dealing with Uncertainty
Dealing with uncertainty is a fundamental issue in forecasting. Forecasts often rely on imperfect or incomplete data, which leads to potential errors and variability in predictions. Uncertainty can arise from various sources, making it essential to address these points systematically to enhance the reliability of the forecasting models.
Measurement Errors
Measurement errors play a pivotal role in the uncertainties involved when constructing forecasting models. These errors can stem from various factors such as incorrect data entry, limitations in measuring tools, or insufficient data collection processes. The significance of recognizing and mitigating measurement errors lies in their potential to distort the results of the analysis. \
The key characteristic of measurement errors is that they can be systematic or random. \ Systematic errors can introduce bias, which can mislead decision-makers. Therefore, understanding the nature of these errors allows for adjusting forecasts to minimize their impact. A benefit of addressing measurement errors is the opportunity to refine data collection techniques, thus improving the quality of future data.
However, it is equally important to note that measurement errors also present disadvantages. They could lead to unnecessary complexity in models if every potential error source is accounted for.
Model Assumptions
Model assumptions also contribute significantly to the overall effectiveness of forecasting methods. These assumptions are foundational elements that support the theoretical framework of a model. Assumptions can simplify real-world complexities, making analysis easier and quicker. However, faulty assumptions can lead to significant miscalculations or misleading conclusions.
A primary advantage of model assumptions is that they create a structured approach to understanding data relationships. For example, common assumptions like linearity or independence facilitate the design of statistical models. Nevertheless, a key downside is that if the assumptions do not hold true for a given dataset, it can severely affect the outcomes, leading to overconfident and inaccurate predictions.
Overfitting and Underfitting
The concepts of overfitting and underfitting are crucial to grasp for anyone engaging in forecasting efforts. Both phenomena can derail the integrity of model outcomes if not correctly managed.
Overfitting occurs when a model is excessively complex, capturing noise along with the actual patterns in the data. It tends to show excellent performance on training data but often fails on new, unseen data. On the other hand, underfitting happens when a model is too simple. It cannot capture important characteristics of the data, leading to poor performance as well.
To optimize forecasting methods, practitioners must find a balance between overfitting and underfitting. Techniques such as cross-validation can aid in determining an optimal level of model complexity that maintains solid predictive power without succumbing to these common pitfalls.
Improving Forecasting Accuracy
Improving forecasting accuracy is vital in ensuring that predictions made using forecasting equations are reliable and actionable. Accurate forecasts are not only essential for strategic decision-making but also for resource allocation and risk management. Various factors contribute to forecasting accuracy, including the quality of data, the models used, and the methods of analysis. By focusing on improving accuracy, researchers and practitioners can minimize errors and better interpret the underlying trends in their data.
Incorporating Advanced Techniques
Machine Learning Approaches
Machine learning approaches represent a powerful method of enhancing forecasting accuracy. These techniques rely on algorithms that learn patterns from historical data, adjusting predictions based on new data inputs. The key characteristic of machine learning is its ability to handle large datasets and identify complex relationships that traditional methods may overlook. This makes it a popular choice in numerous scientific disciplines.
A unique feature of machine learning approaches is their adaptability. As data evolves, models can be retrained, ensuring that forecasts remain relevant. The advantages include improved accuracy, especially in nonlinear scenarios, and the ability to automate the modeling process, saving time and resources. However, potential disadvantages should not be ignored. These include the risk of overfitting, where a model learns noise rather than the signal, leading to poor generalization to new data.
Time Series Analysis
Time series analysis is another critical technique in improving forecasting accuracy. This method analyzes data points collected or recorded at specific time intervals to identify trends, cycles, and seasonality. The key characteristic of time series analysis is its capability to predict future values based on past observations. This method suits many applications, particularly those where temporal factors significantly influence outcomes.
One unique feature of time series analysis is its emphasis on temporal structure, allowing practitioners to project future events based on historical trends. It provides clear benefits such as capturing seasonality and cyclical patterns in the data. Nonetheless, challenges exist, including the assumption that past patterns will continue into the future, which may not always hold true in rapidly changing environments.
Regular Updates and Revisions
Regular updates and revisions to forecasting models are crucial for maintaining accuracy over time. As new data becomes available or as conditions change, it is essential to revisit and adjust existing models. This process ensures that the forecasts remain relevant and reliable, reflecting the most current information.
Incorporating routine revisions can enhance the overall effectiveness of forecasting efforts, providing an effective strategy to tackle the uncertainty often encountered in predictive modeling.
Ending and Future Directions
The section on conclusion and future directions holds critical significance within this article. It synthesizes the diverse elements discussed throughout, providing coherence and closure to the complex topic of forecasting equations. Many practitioners and scholars tend to overlook this segment, yet it encapsulates the essence of understanding today's methodologies while paving the way for future exploration.
A well-structured conclusion highlights the importance of grasping the principles of forecasting. This includes learning not just about the theory and statistics, but also recognizing how these elements converge in practical applications. Such understanding is beneficial for enhancing predictive accuracy across various fields.
Summary of Key Points
This section provides a recap of the foundational aspects covered earlier in the article. Key points include:
- Definition and Importance: Forecasting equations are essential in predicting outcomes, making informed decisions, and strategic planning.
- Theoretical Framework: Includes the types of forecasting, highlighting qualitative and quantitative methods.
- Mathematical Foundations: Focuses on dependent and independent variables that form the core of the forecasting models.
- Techniques: Covers data collection methods, model selection, and evaluation frameworks.
- Applications: Reviews how forecasting is utilized in biology, economics, and meteorology.
- Challenges: Discusses the uncertainties and issues like overfitting that practitioners face.
- Improvements: Offers insights into advanced techniques like machine learning that enhance model precision.
Emerging Trends in Forecasting Research
In the ever-evolving landscape of forecasting research, several trends have emerged that warrant attention. These include:
- AI and Machine Learning Integration: The application of artificial intelligence is redefining how forecasting models can learn from vast datasets.
- Focus on Real-time Data: The shift towards utilizing real-time data for immediate insights is gaining traction, enhancing the dynamism of forecasts.
- Sustainability Considerations: Increasing focus on how forecasting impacts sustainability and decision-making processes in environmental contexts.
- Customized Models: Shift towards customizing forecasting models to specific industrial needs rather than one-size-fits-all solutions.
As these trends reshape the forecasting landscape, future research should continue to explore these developments. By staying abreast of new methodologies and adapting to an increasingly complex data environment, researchers can enhance the reliability and applicability of forecasting equations. The future of forecasting research promises to be dynamic, requiring ongoing adaptation and innovation.